Abstract
The brain’s remarkable ability to learn and execute various motor behaviours harnesses the capacity of neural populations to generate a variety of activity patterns. Here we explore systematic changes in preparatory activity in motor cortex that accompany motor learning. We trained rhesus monkeys to learn an arm-reaching task1 in a curl force field that elicited new muscle forces for some, but not all, movement directions2,3. We found that in a neural subspace predictive of hand forces, changes in preparatory activity tracked the learned behavioural modifications and reassociated4 existing activity patterns with updated movements. Along a neural population dimension orthogonal to the force-predictive subspace, we discovered that preparatory activity shifted uniformly for all movement directions, including those unaltered by learning. During a washout period when the curl field was removed, preparatory activity gradually reverted in the force-predictive subspace, but the uniform shift persisted. These persistent preparatory activity patterns may retain a motor memory of the learned field5,6 and support accelerated relearning of the same curl field. When a set of distinct curl fields was learned in sequence, we observed a corresponding set of field-specific uniform shifts which separated the associated motor memories in the neural state space7,8,9. The precise geometry of these uniform shifts in preparatory activity could serve to index motor memories, facilitating the acquisition, retention and retrieval of a broad motor repertoire.
This is a preview of subscription content
Access options
Subscribe to Journal
Get full journal access for 1 year
199,00 €
only 3,90 € per issue
Tax calculation will be finalised during checkout.
Buy article
Get time limited or full article access on ReadCube.
$32.00
All prices are NET prices.
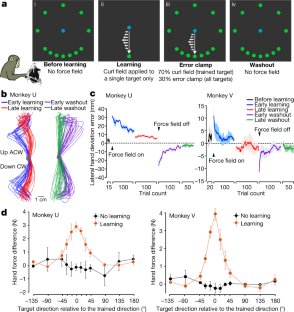
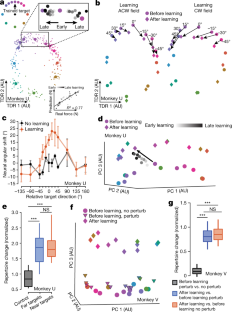
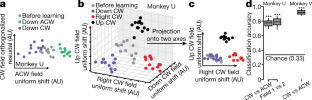
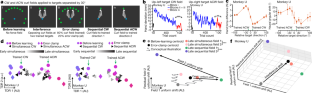
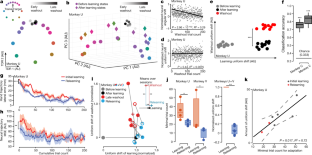
Data availability
The data that support the findings of the current study are available from the corresponding authors upon reasonable request. Source data are provided with this paper.
Code availability
The code for the repertoire change analysis is available on github (https://github.com/mattgolub/bci_learning). The code for the TDR and uniform-shift analyses is available on github (https://github.com/xlsun79/TDRandUniformShiftAnalyses). All other code is available from the corresponding author upon reasonable request.
References
- 1.
Shadmehr, R. & Mussa-Ivaldi, F. A. Adaptive representation of dynamics during learning of a motor task. J. Neurosci. 14, 3208–3224 (1994).
- 2.
Shadmehr, R. Generalization as a behavioral window to the neural mechanisms of learning internal models. Hum. Mov. Sci. 23, 543–568 (2004).
- 3.
Howard, I. S. & Franklin, D. W. Neural tuning functions underlie both generalization and interference. PLoS ONE 10, e0131268 (2015).
- 4.
Golub, M. D. et al. Learning by neural reassociation. Nat. Neurosci. 21, 607–616 (2018).
- 5.
Li, C. S., Padoa-Schioppa, C. & Bizzi, E. Neuronal correlates of motor performance and motor learning in the primary motor cortex of monkeys adapting to an external force field. Neuron 30, 593–607 (2001).
- 6.
Xiao, J. Premotor neuronal plasticity in monkeys adapting to a new dynamic environment. Eur. J. Neurosci. 22, 3266–3280 (2005).
- 7.
Sheahan, H. R., Franklin, D. W. & Wolpert, D. M. Motor planning, not execution, separates motor memories. Neuron 92, 773–779 (2016).
- 8.
Sheahan, H. R., Ingram, J. N., Žalalytė, G. M. & Wolpert, D. M. Imagery of movements immediately following performance allows learning of motor skills that interfere. Sci. Rep. 8, 14330 (2018).
- 9.
Schween, R., Langsdorf, L., Taylor, J. A. & Hegele, M. How different effectors and action effects modulate the formation of separate motor memories. Sci. Rep. 9, 17040 (2019).
- 10.
Krakauer, J. W., Hadjiosif, A. M., Xu, J., Wong, A. L. & Haith, A. M. Motor learning. Compr. Physiol. 9, 613–663 (2019).
- 11.
Thoroughman, K. A. & Shadmehr, R. Learning of action through adaptive combination of motor primitives. Nature 407, 742–747 (2000).
- 12.
Herzfeld, D. J., Vaswani, P. A., Marko, M. K. & Shadmehr, R. A memory of errors in sensorimotor learning. Science 345, 1349–1353 (2014).
- 13.
Howard, I. S., Wolpert, D. M. & Franklin, D. W. The effect of contextual cues on the encoding of motor memories. J. Neurophysiol. 109, 2632–2644 (2013).
- 14.
Shenoy, K. V., Sahani, M. & Churchland, M. M. Cortical control of arm movements: a dynamical systems perspective. Annu. Rev. Neurosci. 36, 337–359 (2013).
- 15.
Vyas, S., Golub, M. D., Sussillo, D. & Shenoy, K. V. Computation through neural population dynamics. Annu. Rev. Neurosci. 43, 249–275 (2020).
- 16.
Sauerbrei, B. A. et al. Cortical pattern generation during dexterous movement is input-driven. Nature 577, 386–391 (2020).
- 17.
Stavisky, S. D., Kao, J. C., Ryu, S. I. & Shenoy, K. V. Trial-by-trial motor cortical correlates of a rapidly adapting visuomotor internal model. J. Neurosci. 37, 1721–1732 (2017).
- 18.
Vyas, S. et al. Neural population dynamics underlying motor learning transfer. Neuron 97, 11771186.e3 (2018).
- 19.
Perich, M. G., Gallego, J. A. & Miller, L. E. A neural population mechanism for rapid learning. Neuron 100, 964–976.e7 (2018).
- 20.
Vyas, S., O’Shea, D. J., Ryu, S. I. & Shenoy, K. V. Causal role of motor preparation during error-driven learning. Neuron 106, 329–339.e4 (2020).
- 21.
Churchland, M. M. et al. Neural population dynamics during reaching. Nature 487, 51–56 (2012).
- 22.
Mante, V., Sussillo, D., Shenoy, K. V. & Newsome, W. T. Context-dependent computation by recurrent dynamics in prefrontal cortex. Nature 503, 78–84 (2013).
- 23.
Even-Chen, N., Sheffer, B., Vyas, S., Ryu, S. I. & Shenoy, K. V. Structure and variability of delay activity in premotor cortex. PLoS Comput. Biol. 15, e1006808 (2019).
- 24.
Arce, F., Novick, I., Mandelblat-Cerf, Y. & Vaadia, E. Neuronal correlates of memory formation in motor cortex after adaptation to force field. J. Neurosci. 30, 9189–9198 (2010).
- 25.
Donchin, O., Francis, J. T. & Shadmehr, R. Quantifying generalization from trial-by-trial behavior of adaptive systems that learn with basis functions: theory and experiments in human motor control. J. Neurosci. 23, 9032–9045 (2003).
- 26.
Jarosiewicz, B. et al. Functional network reorganization during learning in a brain-computer interface paradigm. Proc. Natl Acad. Sci. USA 105, 19486–19491 (2008).
- 27.
Chase, S. M., Kass, R. E. & Schwartz, A. B. Behavioral and neural correlates of visuomotor adaptation observed through a brain–computer interface in primary motor cortex. J. Neurophysiol. 108, 624–644 (2012).
- 28.
Oby, E. R. et al. New neural activity patterns emerge with long-term learning. Proc. Natl Acad. Sci. USA 116, 15210–15215 (2019).
- 29.
Sakellaridi, S. et al. Intrinsic variable learning for brain–machine interface control by human anterior intraparietal cortex. Neuron 102, 694–705.e3 (2019).
- 30.
Hwang, E. J., Bailey, P. M. & Andersen, R. A. Volitional control of neural activity relies on the natural motor repertoire. Curr. Biol. 23, 353–361 (2013).
- 31.
Rokni, U., Richardson, A. G., Bizzi, E. & Seung, H. S. Motor learning with unstable neural representations. Neuron 54, 653–666 (2007).
- 32.
Franklin, D. W., Osu, R., Burdet, E., Kawato, M. & Milner, T. E. Adaptation to stable and unstable dynamics achieved by combined impedance control and inverse dynamics model. J. Neurophysiol. 90, 3270–3282 (2003).
- 33.
Heald, J. B., Franklin, D. W. & Wolpert, D. M. Increasing muscle co-contraction speeds up internal model acquisition during dynamic motor learning. Sci. Rep. 8, 16355 (2018).
- 34.
Richardson, A. G., Borghi, T. & Bizzi, E. Activity of the same motor cortex neurons during repeated experience with perturbed movement dynamics. J. Neurophysiol. 107, 3144–3154 (2012).
- 35.
Churchland, M. M., Cunningham, J. P., Kaufman, M. T., Ryu, S. I. & Shenoy, K. V. Cortical preparatory activity: representation of movement or first cog in a dynamical machine? Neuron 68, 387–400 (2010).
- 36.
Russo, A. A. et al. Motor cortex embeds muscle-like commands in an untangled population response. Neuron 97, 953–966.e8 (2018).
- 37.
Ames, K. C., Ryu, S. I. & Shenoy, K. V. Neural dynamics of reaching following incorrect or absent motor preparation. Neuron 81, 438–451 (2014).
Acknowledgements
We thank the members of the Shenoy laboratory at Stanford University for comments and discussions on the methods and results; T. Fisher for help with experiments; M. Risch, M. Wechsler and R. Reeder for expert veterinary care; B. Davis for administrative support; and W. L. Gore for donating Preclude artificial dura used as part of the chronic electrode array implantation procedure. X.S. was supported by a Stanford Interdisciplinary Graduate Fellowship, a Stanford Bio-X Honorary Fellowship and Stanford Department of Biology Funding. D.J.O. was supported by a US National Science Foundation graduate research fellowship and a Stanford Graduate Fellowship. M.D.G. was supported by an NIH K99/R00 award NIMH-K99MH121533. E.M.T. was supported by an NIH NRSA grant 1F31NS089376-01, a Stanford Graduate Fellowship and an NSF IGERT grant 0734683. S.V. was supported by an NIH F31 Ruth L. Kirschstein National Research Service Award 1F31NS103409-01, an NSF Graduate Research Fellowship and a Ric Weiland Stanford Graduate Fellowship. K.V.S. was supported by the following awards: NIH Director’s Pioneer Award 8DP1HD075623, Defense Advanced Research Projects Agency (DARPA) Biological Technology Office (BTO) ‘NeuroFAST’ award W911NF-14-2-0013, the Simons Foundation Collaboration on the Global Brain awards 543045, the Office of Naval Research N000141812158 and the Howard Hughes Medical Institute.
Author information
Affiliations
Contributions
X.S. conceived the project and designed the experiments with input from D.J.O. and M.D.G. X.S. conducted the V-probe, Utah array and EMG recordings. X.S. and D.J.O. conducted the Neuropixels recordings, with extensive assistance from E.M.T. S.V. collected the VMR data. X.S. performed data analysis with significant assistance from D.J.O. and M.D.G., as well as input from S.V. S.I.R. led the recording chamber and array implantation surgeries. X.S. and D.J.O. wrote the manuscript with input and editing from all authors. K.V.S. was involved with all aspects of the research.
Corresponding authors
Ethics declarations
Competing interests
K.V.S. is a consultant to Neuralink Corp. and CTRL-Labs Inc., in the Reality Labs Division of Meta (formerly FaceBook). He is on the Scientific Advisory Boards of Inscopix Inc., Mind X Inc. and Heal Inc. These entities did not support or influence this work. The authors declare no other competing interests.
Peer review information
Nature thanks Aaron Batista, J. Andrew Pruszynski and the other, anonymous reviewers for their contribution to the peer review of this work. Peer review reports are available.
Additional information
Publisher’s note Springer Nature remains neutral with regard to jurisdictional claims in published maps and institutional affiliations.
Extended data figures and tables
Extended Data Fig. 1 Additional information for task design, recording sites and behavioural performance.
a, Spatial arrangements of the 12 reaching targets. The target density near the trained target (down, up or right) was higher in order to sample more neural states for reaches that were more likely to be altered by learning, for the purpose of studying generalization of learning3. Ideally, one would like to have equally-spaced reach targets as dense as possible, but because monkeys could perform a limited number of trials each day, a compromise solution was to increase the density of targets near the trained target. Note that the curl field can be either clockwise (CW) or counterclockwise (CCW). Here we show CW fields as an example. b, Utah-array implant locations in monkey U and recording sites in monkey V. Recordings were performed in PMd and M1 in the hemisphere contralateral to the reaching arm. Top panel: an intraoperative photo of three Utah-array implants in monkey U. Bottom panel: a schematic map illustrating the approximate locations of recording sites in monkey V based on stereotactic coordinates; data in this work included units recorded from multi-electrode V-probes and Neuropixels probes. Histology has not yet been done on either monkey. Using the cortical landmarks, we estimated that the recording sites in monkey V largely overlapped with the lateral half of the area covered by the three Utah arrays in monkey U. AS: Spur of arcuate. CS: central sulcus. PCD: precentral dimple. c, We computed behavioural (top panel) and neural generalization (bottom panel) with the sign of the effects flipped for CCW fields to match the effects of CW fields, compared to Fig. 1d and Fig. 2c. We found a spatial asymmetry in behavioural and neural generalization, with more learning in the ‘push’ direction (i.e., the direction to oppose the curl field). Error bars, s.e.m. across sessions (monkey U, n = 4, 3; monkey V, n = 5, 3). d, Top panel: trial-averaged hand speed in different blocks over multiple learning sessions. Shaded area, s.e.m. across sessions (monkey U, n = 4; monkey V, n = 5). Bottom panel: compensatory hand force perpendicular to the reach direction in one example session. Hand force in late-learning trials (dark red) showed a more stereotypical, less variable temporal pattern with an earlier onset than in early-learning trials (light red). Time zero, movement onset.
Extended Data Fig. 2 Additional results of neural activity patterns during curl field learning and VMR learning.
a, Initial hand forces predicted by the 2D TDR preparatory states were correlated with real forces of the upcoming movement (the slope is 0.41 and the intercept is 0.02 with \(}^{2}\) = 0.59 and P = 6.06 x 10−9). The sign of hand force indicates its direction. Lighter dots, earlier learning trials; darker dots, later learning trials. b, Single-trial prediction MSE of initial hand forces was significantly smaller using original data than shuffled data (two-sided Wilcoxon rank-sum test: P = 0.0006 for both training and test sets of monkey U and P = 0.008 for both training and test sets of monkey V). Training set: before-learning trials. Test set: learning trials. Control results (blue) were forces predicted by models built from training sets that had neural and behavioural data shuffled. One datapoint per session. c, Changes of preparatory states in the force-predictive TDR subspace reflected generalization of learning, quantified as the rotatory angle from before-learning to error-clamp neural states. Zero degree on the x axis, the trained target. Error bars, s.e.m. across sessions (n = 5, 3). d, Normalized single-trial neural shift during learning along the uniform-shift learning axis. Solid line: linear-log regression (n = 1200, 900). e, Preparatory neural repertoires changed similarly for trained and untrained reaches. Black: no-learning control results (n =36). Blue: far targets more than 45 degrees from the trained target (n = 15). Red: near targets within 45 degrees from the trained target (n = 21). One-sided Wilcoxon rank-sum test: Pblack vs. blue = 2.33 x 10−7, Pblack vs. red = 4.74 x 10−8, Pblue vs. red = 0.059. f, g, VMR learning results. f, Preparatory neural states projected to PCs 1-3. After-learning states (diamonds) were mixed with before-learning states (circles). One example session. g, Preparatory and peri-movement neural activity patterns did not show repertoire change during VMR learning. One-sided Wilcoxon rank-sum test: P > 0.1 for all comparisons. Three learning sessions (n = 24) and three control sessions (n = 24) for both monkeys. h, i, Neural preparatory states in the 3D TDR subspace. The 3D subspace was constructed by TDR capturing the variance due to initial hand forces and a binary indicator of trial conditions (an indicator of before-learning versus after-learning). One example session. h, In the force-predictive TDR subspace, rotatory shifts of preparatory neural states were similar to Fig. 2b. i, Along the TDR 3 axis (the binary indicator axis), this 3D model revealed a uniform shift similar to what we observed along PC 3 in the PCA subspace (Fig. 2d). For all the box plots, the central line indicates the median, the bottom and top edges indicate the 25th and 75th percentiles of the data, and the whiskers extend to the 5th and 95th percentiles of the data.
Extended Data Fig. 3 Relationships between neural population dimensions and total neural variance explained by different dimensions.
a, Pairwise dot products between neural population dimensions. Values close to 1 indicate that two dimensions are closely aligned and values close to 0 indicate that two dimensions are nearly orthogonal. In each session, we calculated the dot product of TDR 1 and PC 1 and the dot product of TDR 1 and PC 2, and took the larger value of the two dot products (TDR 1 vs. PC 1 / 2). We then calculated the dot product of TDR 2 and the PC axis not used for multiplying with TDR 1 (TDR 2 vs. PC 1 / 2). The PC 1 / 2 plane largely overlapped with the TDR 1 / 2 plane (black). The TDR 1, TDR 2, and hand-speed TDR axes were all nearly orthogonal to the uniform-shift learning axis (blue and red). PC 3 largely overlapped with the uniform-shift learning axis (yellow). Two-sided Wilcoxon rank-sum test: monkey U, **P = 4.04 x 10−3, *P = 0.029; monkey V, ***P = 6.66 x 10−4, **P = 7.94 x 10−3. Right panel: a schematic illustration of projecting data points from axis \(\alpha \) to axis \(\beta \) and the corresponding dot product. b, c, The portion of total neural activity variance explained by the TDR 1 and TDR 2 (hand force) axes, hand-speed TDR axis, uniform-shift axis and PCs 1-3. b, Two-sided Wilcoxon rank-sum test: monkey U, *P = 0.029, n.s. P = 1; monkey V, *P = 0.016 and 0.032, n.s. P = 0.42. a–c, n = 4 (monkey U) and n = 5 (monkey V). For all the box plots, the central line indicates the median, the bottom and top edges indicate the 25th and 75th percentiles of the data, and the whiskers extend to the 5th and 95th percentiles of the data.
Extended Data Fig. 4 EMG signals of 6 upper limb muscles (bicep, radialis flexor, radialis extensor, pectoralis, posterior deltoid, lateral deltoid).
Time zero, movement onset. One example condition (CW curl field applied to down reaches). Shaded area, s.e.m. across trials. a, EMG signals in before-learning, late-learning, error-clamp and late-washout blocks. Muscle activity did not show signs of muscle co-contraction during learning (red). Muscle activity during the preparatory period remained flat and around the same level across all blocks (two-sided rank-sum test: P < 0.0001 for comparing late-learning or error-clamp bicep activity with before-learning or late-washout bicep activity during the preparatory period; P > 0.3 for all the other pairs of comparison during the preparatory period). Muscle activity patterns in before-learning (black) and late-washout trials (blue) were very similar. Muscle activity patterns in late-learning (red) and error-clamp trials (purple) were very similar. b, EMG signals in before-learning (black) and error-clamp (purple) blocks did not show a uniform shift across all 12 reaching targets. For all six muscles, EMG activity after learning increased in some directions and decreased in other directions. Muscle activity of reaching to the target 135 degrees away from the trained target (i.e., far targets with almost no behavioural generalization, see Fig. 1c) in before-learning and error-clamp trials showed similar temporal patterns.
Extended Data Fig. 5 The uniform shift is not due to the error clamp.
a, Preparatory states in error-clamp (diamonds) and no-clamp (circles) trials were not significantly different in no-learning control sessions (Hotelling’s T2 test: P > 0.5 for all control sessions of monkeys U and V). One example session is shown. b, In the error-clamp block (block iii) of learning sessions, the late-learning preparatory state and error-clamp state of the trained target were not significantly different (Hotelling’s T2 test: P > 0.05 for all learning sessions of monkey U and P > 0.1 for all learning sessions of monkey V). Arrows point to the before-learning state (purple circle) and error-clamp state (purple diamond) of the trained target. One example session is shown. c, Late-learning preparatory states comprised a new neural repertoire following learning but not during control reaches with random force perturbations. One-sided Wilcoxon rank-sum test: ***P = 1.83 x 10−5, n.s. P = 0.99 (n = 12 per box). The results are similar to Fig. 2g where we used after-learning, error-clamp trials to compute the repertoire change. d–f, The uniform shift was not due to reorientation of the TDR plane in error-clamp trials. d, TDR axes using only error-clamp trials (TDR-EC) and TDR axes using only before-learning trials (TDR-BL) were largely aligned. The error-clamp TDR 1 axis and before-learning TDR 1 axis were highly aligned, and same for TDR 2 axes (black). The error-clamp TDR axes were nearly orthogonal to the uniform-shift axis (red), similar to the before-learning TDR axes shown in Extended Data Fig. 3a. One-sided Wilcoxon rank-sum test: monkey U, n = 4 and *P = 0.014; monkey V, n = 5 and **P = 4.0 x 10−3. e, In the interference experiments, the force-predictive TDR planes constructed from only before-learning (BL) trials, only error-clamp (EC) trials, and both (BL+EC) were significantly aligned. One-sided signed-rank test comparing dot products to 0.8: n = 8 from monkeys U + V, **P = 3.9 x 10−3. f, In the interference experiments, preparatory neural states showed similar patterns in the force-predictive TDR plane built from only before-learning (BL) trials, only error-clamp (EC) trials, or both (BL+EC). One example session. Small black arrows point to the before-learning states of trained targets. For all the box plots, the central line indicates the median, the bottom and top edges indicate the 25th and 75th percentiles of the data, and the whiskers extend to the 5th and 95th percentiles of the data.
Extended Data Fig. 6 Stability of multi-session recordings and the geometric relationship between uniform-shift axes for learning multiple curl fields.
a, b, Spike waveforms and peristimulus time histograms (PSTHs) of three example neurons across sessions. The same 71 neurons from monkey U Utah-array recordings were selected post-hoc by comparing waveform correlations and tracked over five successive sessions. a, All selected neurons had nearly-identical waveforms. b, Like the three example neurons, most selected neurons had similar direction-tuning properties for before-learning reaches across sessions. Go, go cue. Move, movement onset. c, Distribution of dot products between uniform-shift axes for learning two opposite fields applied at one reach target sequentially (green) or two distinct fields applied at different reach targets sequentially (purple). We compared them with simulated distributions of dot products between uniform shifts predicted by ‘orthogonal’ (red, around 0), ‘parallel’ (blue, around 1) and ‘antiparallel’ (black, around -1) relationships (see Measurement of geometric relationships between uniform-shift axes in Methods). Top inset, the zoom-in view of each simulated distribution.
Extended Data Fig. 7 Interference and sequential learning of opposite curl fields applied at two targets 30 degrees apart, monkey V results.
a, Behavioural learning quantified by lateral hand deviation. Lateral hand deviation in both curl fields decreased slowly during simultaneous learning (blue) and further reduced during sequential learning (red). One-sided Wilcoxon rank-sum test: PCCW = 1.30 x 10−10, PCW = 1.51 x 10−11. b, Behavioural and neural generalization of simultaneously learning two fields. Behavioural generalization was measured by perpendicular hand force differences between error-clamp and before-learning trials, and neural generalization was measured by the rotatory angle between before-learning and error-clamp neural states. Zero degree on the x axis, the middle target between the two trained targets. Error bars, s.e.m. from resampling (100 repeats). c, Preparatory neural states in the force-predictive TDR subspace. Before-learning states (circles) spatially organized corresponding to reach directions. Error-clamp states (diamonds) rotated counterclockwise for reach targets near the CW field and clockwise for targets near the CCW field. Preparatory states of the two trained targets (triangles) rotated opposite their curl field directions in blocks ii and iii (left panel), and further separated in the sequential-learning block (right panel). Small yellow arrows point to the before-learning states of trained targets. Neural states of seven nearest targets are visualized in c, and quantified neural changes for all 11 targets are shown in b. d, Left panel: preparatory activity projected into the subspace spanned by the two field-specific uniform shifts. Without orthogonalization, these two uniform shifts were 128 degrees apart. The uniform shifts were orthonormalized before projection such that: x axis = field 1 uniform shift, y axis = field 2 uniform shift – the projection of field 2 uniform shift on field 1 uniform shift. During simultaneous learning (blocks ii and iii), preparatory states of each field (orange and pink) moved in its specific uniform-shift direction while also progressing in the other uniform-shift direction, and were significantly separated (Hotelling’s T2 test: P = 2.58 x 10−6). Grey arrows illustrate the hypothesized trial-by-trial progression of preparatory states for both fields during simultaneous learning. During sequential learning, preparatory states of each field (green and purple) further separated (Hotelling’s T2 test: P = 0). Right panel: A residual interference shift orthogonal to the field-specific uniform shifts occurred during simultaneous learning. Neural states in the left panel are the projection of neural states into the grey plane in the right panel.
Extended Data Fig. 8 Interference and sequential learning of opposite curl fields applied to the same target.
a, Block design of the interference experiment. Same as in Fig. 4a except that two opposite curl fields were applied to the same reach target. b, Behavioural learning quantified by lateral hand deviation. Hand lateral deviation in both curl fields only slightly decreased during simultaneous learning (blue), and significantly reduced during sequential learning (red). One-sided rank-sum test: monkey U, ***P = 1.51 x 10−11; monkey V, ***P = 1.51 x 10−11. c, Preparatory neural states in the force-predictive TDR subspace. In blocks ii and iii (left panel), preparatory states of the two curl fields (triangles) were mixed together around the before-learning state (circle). Error-clamp states (diamonds) of most targets shifted from their corresponding before-learning states. These shifts did not show coherent patterns across targets or monkeys and were likely unrelated to learning. In the sequential-learning block (right panel), preparatory states of the two curl fields (triangles) gradually rotated opposite their curl field directions. The small arrow points to the before-learning state of the reach target that later had curl fields (trained target). Neural states for seven nearest targets are shown. d, Perpendicular hand force differences between error-clamp and before-learning trials (top panel), and the rotatory angle from before-learning to error-clamp neural states (bottom panel), did not show coherent patterns across targets or monkeys. Zero degree on the x axis, the trained target. Error bars, s.e.m. from resampling (100 repeats). e, Uniform shifts for learning two curl fields and the residual interference shift were defined in the same way as in Fig. 4e, f. The two field-specific uniform shifts were close to antiparallel (monkey U, dot product = - 0.79; monkey V, dot product = -0.64), and so we could visualize preparatory neural states in a 2D plane spanned by the field 1 uniform shift and the residual interference shift. In blocks ii and iii, preparatory states of the two curl fields (orange and pink) shifted away from the before-learning centroid (grey circle) along the residual interference axis, but they remained close to each other (Hotelling’s T2 test: monkey U, P = 0.66; monkey V, P = 0.98). During sequential learning, preparatory states of the two curl fields (green and purple) were separated by opposite uniform shifts (Hotelling’s T2 test: monkey U, P = 2.49 x 10−4; monkey V, P = 2.90 x 10−5). b–e, One session per monkey. Though just one session of this interference experiment was performed with each monkey, the results were consistent across monkeys and complimentary to findings when monkeys learned multiple fields sequentially, which supported the indexing hypothesis (Fig. 3).
Extended Data Fig. 9 Monkey V washout results and additional information on the neural population correlate of motor memory retention.
a, The angular difference between washout states and the before-learning state gradually decreased on a single-trial basis (grey dots: single-trial data points from all sessions; solid line: linear regression). Normalized against the maximum in each session. b, Distance between washout states and the before-learning state along the uniform-shift learning axis did not show a significant trend of increase or decrease (solid line: linear regression). Each dot is a single trial. c, Hand deviation was smaller during relearning than during initial learning (one-sided Wilcoxon rank-sum test: P = 0.0015). d, Neural trajectories approached late-learning trajectories faster during relearning than initial learning. One-sided rank-sum test: P = 6.18 x 10−4. c, d, Shaded area, s.e.m. across sessions (n = 3). e, Distribution of dot products between uniform-shift learning and washout axes (purple), compared to simulated distributions of dot products between uniform-shift axes predicted by orthogonal (red), parallel (blue) and antiparallel (black) relationships. f, Distribution of dot products between uniform-shift axes for learning the same curl field in two sessions 18 days apart (green, close to 1). g, Neural trajectories of before-learning, late-learning, late-washout and late-relearning conditions (−150 to +150 ms from target onset, covered by the grey circle; −50 to +50 ms from the go cue, covered by the grey ellipse; and −200 to +400 ms from movement onset). Movement preparation and execution periods are noted on the trajectories. Black arrows show the direction of neural trajectories. The late-washout trajectory (green) was less similar to the before-learning trajectory (black) during movement preparation than execution. TO: target onset. GC: go cue. One example session. h, During preparatory period (prep), the similarity between late-washout and before-learning neural trajectories was significantly lower than the similarity between before-learning neural trajectories. During movement period (move), the similarity between late-washout and before-learning neural trajectories could compare to the similarity between before-learning neural trajectories. One-sided rank-sum test: seven sessions from monkeys U + V, **P = 0.0012, n.s. P = 0.50. For each box, the central line indicates the median, the bottom and top edges indicate the 25th and 75th percentiles of the data, and the whiskers extend to the 5th and 95th percentiles of the data.
Extended Data Fig. 10 Neural population activity patterns in other time windows.
a, PCs 1-4 during -100 to +100 ms from target onset (TO), -100 to +100 ms from go cue (GC), and -200 to +400 ms from movement onset (MOO). We applied PCA to trial-averaged neural activity for different reach directions in the before-learning and error-clamp blocks. Across all learning sessions in both monkeys, PC 1 explained 30 – 40% of the total variance, PC 2 explained 10 - 20%, PC 3 explained 8 - 10%, and PC 4 explained 6 - 8%. Neural trajectories in PCs 1-4 were bundled together around the target onset time window and diverged around the go cue time window (preparatory period). Error-clamp neural trajectories all shifted from their corresponding before-learning trajectories in PC 3. The time window −50 to +50 ms from go cue (grey shadow) we chose for preparatory neural state analysis was within the preparatory period and had stronger neural tuning than earlier time windows (e.g., the first 100 ms after target onset). Top left inset: color-coded reach directions. b, No significant neural repertoire change of baseline neural activity (before target onset on each trial) after learning the curl field. Black: no-learning control results (n = 36, 36). Red: learning results (n = 48, 36). One-sided Wilcoxon rank-sum test: monkey U, P = 0.999; monkey V, P = 0.595. c–i, Patterns of peri-movement neural population states. c, Peri-movement states of before-learning (color circles), learning (grey circles) and after-learning error-clamp (diamonds) reaches projected to PCs 1-3. After-learning states of the trained target and its nearby untrained targets left the before-learning states. One example session. d, Quantification of neural shift during learning along the ‘peri-movement shift axis’ that connected the before-learning and error-clamp states of the trained target, normalized against the distance between these two states. n = 4 (monkey U), 5 (monkey V). Cuzick’s test: monkey U, P = 0.032; monkey V, P = 3.92 x 10−5. e, The Euclidian distance between before-learning and after-learning peri-movement states showed bell-shaped local generalization. Error bars, s.e.m. across sessions (monkey U: n = 4, 3; monkey V: n = 5, 3). f, Peri-movement activity patterns showed significantly greater repertoire change for the trained target and near targets than far targets (monkey U: n = 28, 20; monkey V: n = 21, 15). Black: no-learning control sessions (n = 36 for both monkeys). One-sided Wilcoxon rank-sum test: monkey U, Pblack vs. blue = 0.26, Pblack vs. red = 4.52 x 10−6, Pblue vs. red = 0.002; monkey V, Pblack vs. blue = 3.70 x 10−7, Pblack vs. red = 6.02 x 10−9, Pblue vs. red = 5.29 x 10−4. g, Peri-movement states in the same PCA subspace during washout. h, Distance between washout and before-learning states decreased significantly along the peri-movement shift axis. Normalized against the distance between the before-learning and after-learning states of the trained target. n = 4 (monkey U), 5 (monkey V). Cuzick’s test: monkey U, P = 0.0077; monkey V, P = 0.0028. i, Pairwise dot products between peri-movement neural dimensions. PCs 1-3 significantly overlapped with the peri-movement shift (one-sided signed-rank test compared to 0: monkeys U + V, n = 9 and P = 0.002 for all comparisons). TDR 1 / 2 axes also significantly overlapped with the peri-movement shift (one-sided signed-rank test compared to 0: monkeys U + V, n = 18 and P = 1.07 x 10−4). For all the box plots, the central line indicates the median, the bottom and top edges indicate the 25th and 75th percentiles of the data, and the whiskers extend to the 5th and 95th percentiles of the data.
Supplementary information
Supplementary Information
This file contains Supplementary Notes 1–3, the Methods and discussion. Supplementary Note 1 contains a brief comparison of changes of initial conditions versus changes of neural dynamics matrix, from the linear dynamical systems perspective. Supplementary Note 2 includes an extensive set of control analyses and results to support the idea that the observed uniform shift did not directly mirror movement output and was learning-related. Supplementary Note 3 contains additional neural results during the peri-movement period that differed from the preparatory neural results. The discussion includes a more detailed discussion of the results.
Supplementary Table 1
Detailed statistics for main figures.
Supplementary Table 2
Variance of the uniform shift explained by multiple alternative models, as discussed in Supplementary Note 2.
Source data
Rights and permissions
About this article
Cite this article
Sun, X., O’Shea, D.J., Golub, M.D. et al. Cortical preparatory activity indexes learned motor memories. Nature (2022). https://ift.tt/3g1tdbb
-
Received:
-
Accepted:
-
Published:
-
DOI: https://ift.tt/3g1tdbb
Comments
By submitting a comment you agree to abide by our Terms and Community Guidelines. If you find something abusive or that does not comply with our terms or guidelines please flag it as inappropriate.
"activity" - Google News
January 26, 2022 at 11:13PM
https://ift.tt/3rMM2V8
Cortical preparatory activity indexes learned motor memories - Nature.com
"activity" - Google News
https://ift.tt/3ddCXMh
https://ift.tt/2WkO13c
Bagikan Berita Ini
0 Response to "Cortical preparatory activity indexes learned motor memories - Nature.com"
Post a Comment